Table of Contents
- What is Retail Analytics?
- Types of Retail Analytics
- Benefits of Retail Analytics
- Here are the 5 main areas to use predictive analytics in retail
What is Retail Analytics?
It’s a broad term used to describe using data, mostly from different sources or systems, to analyze and interpret performance.
In the retail sector, this may involve examining which stores are doing well and not so well, how are online stores performing, and what they should do next to get back on top.
Retail analytics is the new buzzword, but it can be very hard to pin down just what it means.
It’s a broad term used to describe the usage of data for analysis. What’s more, the term “stores” is misleading because it’s just one way to measure performance.
If you sell through different channels, you’ll want to measure them all.
Retail analytics help you understand your customer better so you can segment by behavior or demographics.
4 Types of Retail Analytics
There are 4 types of analytics:
- Descriptive Analytics
- Diagnostic Analytics
- Predictive Analytics
- Prescriptive Analytics
In this post, we shall be talking about predictive analytics and its role in retail. But before that, let’s understand the five forms of retail data analytics, each with its own strengths and weaknesses.
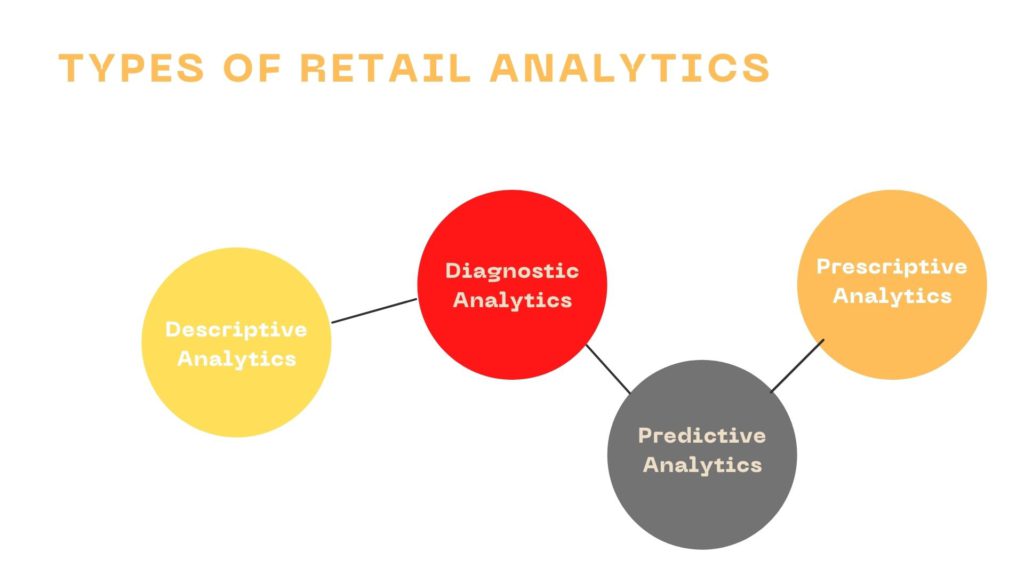
Express Analytics puts the voice of the customer at the heart of the business. Data alone does not drive your business. Decisions do. Speak to Our Experts to get a lowdown on how predictive analytics can help your retail business.
1. Shopper-level analytics: These metrics allow retailers to see how each consumer interacts with each digital or physical channel.
They can pinpoint shoppers’ contact with the brand and find out whether those interactions were positive, negative, or indifferent.
This is the best kind of analytics because it lets retailers engage with customers in real-time. The brands that are seen as most responsive are most likely to gain customers’ loyalty.
2. Transaction-level analytics: These metrics allow retailers to see the impact of marketing campaigns on both purchase intent and shopping behavior.
For example, a retailer might measure the success of an email campaign by counting the number of new customers who buy from that retailer after receiving an email from that retailer.
3. On-shelf analytics: These metrics focus on individual shelf items or categories of goods.
For example, these metrics might track traffic to the Web sites of competing products.
4. Location analytics: These metrics provide a way to see how consumers in a particular geographic area engage with a brand online or off.
For example, in a retail setting, a retailer might track what percentage of shoppers walk in the door and what percentage use a mobile phone to purchase products.
5. Multichannel Analytics: These metrics allow retailers to see how consumers engage with a brand across multiple touchpoints—such as website, store, and mobile.
In a retail setting, this kind of information might help retailers understand how customers engage with a particular product line.
Benefits of Retail Analytics
Retail analytics gives retailers a more complete picture of customer behavior, product usage, and traffic patterns. This kind of information can enable retailers to:
Boost sales by changing the layout of stores, optimizing pricing strategies, or adding new products
Gather valuable insight into which customers are making purchases and with what frequency
Identify geographic areas that need to be targeted for increased marketing efforts
Provide competitive intelligence involving compiling and analyzing data, especially on competitors and the industry as a whole.
For example, retailers might look at their competitors’ websites and analyze what kinds of deals they’re offering and how they’re promoting those deals.
Want to know how Predictive Analytics can help you reach your goals?
5 Main Areas to Use Predictive Analytics in Retail:
While data modeling has been traditionally used extensively in certain industries such as insurance and climate control, the one field where predictive data analytics can be utilized to its full potential is retail.
Retail, more so than any other industry, makes a lot of data.
Unfortunately, that same huge amount of data is also the problem with retail.
With so much data coming in, much of it in real-time, it is difficult to manage, with a lot of that data never getting converted into insights.
To stay ahead of the game in today’s age of e-commerce, retail merchants need to learn how to handle the incoming data and get it ready for analytics.
Any apathy in this means them losing out on one of the most valuable uses of retail analytics – predictive analytics.
Predictive retail analytics can be called the proactive part of retail analytics.
In fact, some consider it to be a ‘crystal ball’ that can accurately tell you what customers may want next. Being able to tell what will happen with your customers can be the difference between dwindling sales and strong revenue.
Before going down that route, however, here’s a list of the kind of data that a retailer needs to have to leverage predictive data analytics:
- Point-of-sale data
- Consumer-related information, including that of loyalty programs
- Consumer demography
- Store and online navigation traffic flow
- Competitive intelligence
- Other external factors such as weather
That certainly seems like a lot. So where does a retailer get all this data from?
- Website
- Smartphone app
- Loyalty programs
- Point-of-sale systems
- Supply chain systems
- In-store sensors & cameras
- Social media networks
So, in which part of their operations can retailers deploy predictive analytics to derive maximum value?
1. Personalization for customers
Understanding customer behavior and combining it with consumer demography is the first step in the deployment of predictive analytics.
Retailers can use it to give targeted and highly customized offers for specific shoppers.
In the past, before data analytics became mainstream, the option of targeted offers was non-existent or was only for large swathes of customers having one or two common characteristics.
But with the emergence of online shopping, and then data analytics, it is now possible to track behavior across channels, i.e. monitor a shopper who researches in the digital store and then goes ahead and purchases the item in the physical store.
Such insights coupled with retail predictive analytics now give merchants the option to make highly personalized offers to customers at a very granular level.
For example, retailers can personalize the in-store experience by giving offers to incentivize frequent buying to drive more purchases, thereby achieving higher sales across all channels.
This technique can be used to upsell or even cross-sell. For example, based on his previous buying history, we know John Doe has a fondness for buying brand X of chocolates at the start of every month.
Using predictive analytics, a retailer can now offer John a buy two get one free deal on chocolate. Considering how consistent his buying behavior is, John will likely take advantage of this coupon, leading to more profit for the company.
2. Inventory and supply chain management
One area which is often neglected is the back-office operations.
Poorly maintained inventory is every retailer’s nightmare. Supply chains need to be optimized to increase operational efficiency.
Predictive analytics helps answer questions such as what to store when to store, and what and when to discard.
Stocking up on slow-moving products or running out of popular ones are both problems. Such insights optimize performance and reduce costs.
Thus, predictive retail analytics removes this uncertainty or any purchase simply based on a hunch.
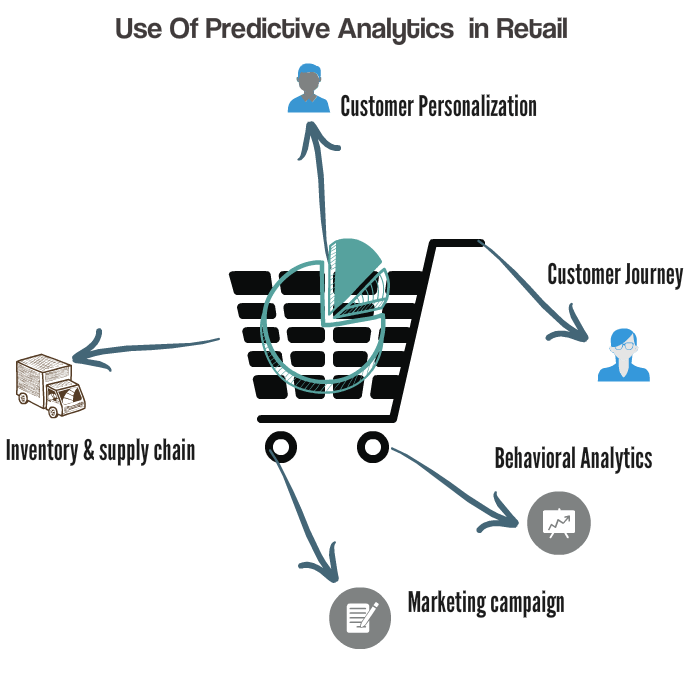
3. Customer segmentation & customer journey
A customer’s journey is a map that tracks the buyer’s experience.
It starts when the customer first makes contact with a brand and ends with a purchase order. The journey traces the process of engagement.
Contrary to popular belief, customer mapping does not end with the client placing an order.
It’s also about a long-term relationship, trying to map the behavior of a customer after he has received his product.
The customer is at the center of every B2C and B2B company, and a map of the customer’s journey gives managers a ringside view of how customers or leads have moved through the sales funnel.
Data-driven insights can help retailers understand each customer’s profile and history across channels.
You can monitor customer activity to determine who your best customers are, and how they and good customers like them, behave and react to your retail marketing.
Predictive Retail analytics helps with not only targeting customers but also their segmentation.
Using affinity analysis, a retailer can cluster the customer base based on common attributes.
Merchants can use response modeling to examine past marketing stimulus and customer response to predict whether using an approach in the future will work.
Churn analysis, on the other hand, tells you the percentage of customers lost over time, as well as the potential revenue lost because of it.
Predictive analytics helps businesses predict a customer’s lifetime value (CLV).
Retailers would like to know how to predict the value of a customer over the course of his/her interactions with their business in the future.
CLV forecasts a discounted value of a customer over time. CLV involves analyzing past behavior to determine the most profitable customers over time.
Want to know how Predictive Analytics can help you reach your goals?
4. Customer behavior or behavioral analytics
People-tracking technology has now made it easy for retailers to find ways of analyzing in-store or online shopping behavior, and assess the impact of merchandising efforts.
Various consumer interaction points can provide data. These include social media, e-commerce sites, credit card swipes (transactions), and so on. Retailers today have access to diverse (and complex) data about their customers.
Using this and even data points captured from earlier marketing and advertising campaigns, retailers can now build predictive models to link past behavior and demographics. Such models aim to score every customer according to the likelihood of them buying certain products.
This entire data-based process also gives retailers invaluable insights into recognizing their high-value customers, establishing the CLV, a customer’s motives behind a purchase, the buying patterns, the preferred channels, and so on.
Retailers armed with such knowledge can Not only throw up personalized offers but also retain new customers. This is reinforced by loyalty programs that encourage them to buy from you over the competition.
But how do you retain those customers who used to be sure things when their loyalty is flagging? Using predictive analytics, retailers can gauge those customers that are drifting, and those that have the potential to be long-term users.
5. Campaign management
Predictive analytics can be used to craft future marketing campaign strategies. The more you know about your customers, the more targeted your messaging can be.
Data-based decision-making reduces how many decisions are based on instincts or guesswork.
CLV can dictate where to focus your ad spend. This method can identify the channels and the times that require an increase in your marketing spending and resources.
To conclude, using data analytics no longer remains the sole purview of the retail biggies such as Amazon.
Thanks to the technology getting cheaper and more mainstream, predictive analytics can now be used even by medium and small retailers to be ahead of the competition.
Your retail business, irrespective of size, now has the ability to unlock unprecedented growth. All you need to do is to tap into our world-class customer data platform Oyster and its AI-powered predictive analytics.
Having your data ducks in a row helps you identify the right audience for your next targeted marketing campaign.
Get in touch to know more
Reference
Predictive Analytics Software in Retail
No comments yet.